
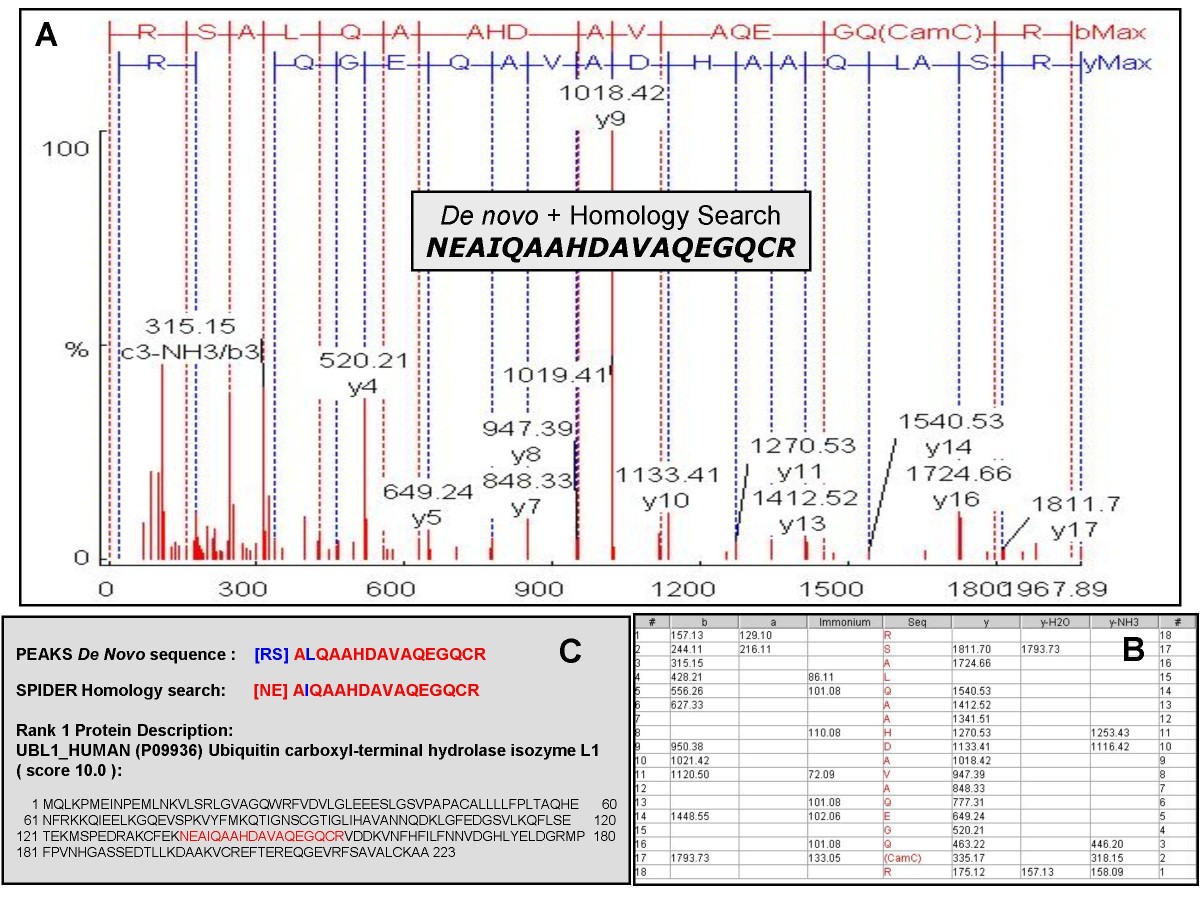
A sequence database also cannot be used for the analysis of some types of immunopeptidomics data, for example, when a phenomenon known as V(D)J recombination results in peptides whose sequences are not encoded in the genome, in antibody sequencing, or in vaccine development when searching for bacterial peptides present on the surface of infected cells. However, relying on a database prevents the detection of unexpected peptide sequences, such as those that arise from genetic variation. Such an approach is often sensible when analyzing samples from a species, such as human, with a well-characterized genome sequence. However, the drawback to any database search methodology is that it requires that we specify a priori which peptides might occur in the sample. The standard method for solving this problem is enumerative, scoring each observed spectrum with respect to a list of candidate peptides (i.e., peptides whose masses are close to the observed precursor mass associated with the spectrum) and reporting the best-scoring peptide-spectrum match (PSM) per spectrum. At the core of this challenge is the spectrum identification problem, in which we are given an observed mass spectrum and the associated mass and charge of the peptide (known as the precursor) that is responsible for generating the spectrum, and we must infer the amino acid sequence of the precursor peptide. Tandem mass spectrometry provides a high-throughput framework for identifying and quantifying proteins in complex biological samples, but determining the exact protein content from observed mass spectra at scale remains a challenge. Casanovo not only achieves superior performance but does so at a fraction of the model complexity and inference time required by other methods. Our experiments show that Casanovo achieves state-of-the-art performance on a benchmark dataset using a standard cross-species evaluation framework which involves testing with out-of-distribution samples, i.e., spectra with never-before-seen peptide labels. We propose a simple yet powerful method for de novo peptide sequencing, Casanovo, that uses a transformer framework to map directly from a sequence of observed peaks (a mass spectrum) to a sequence of amino acids (a peptide).
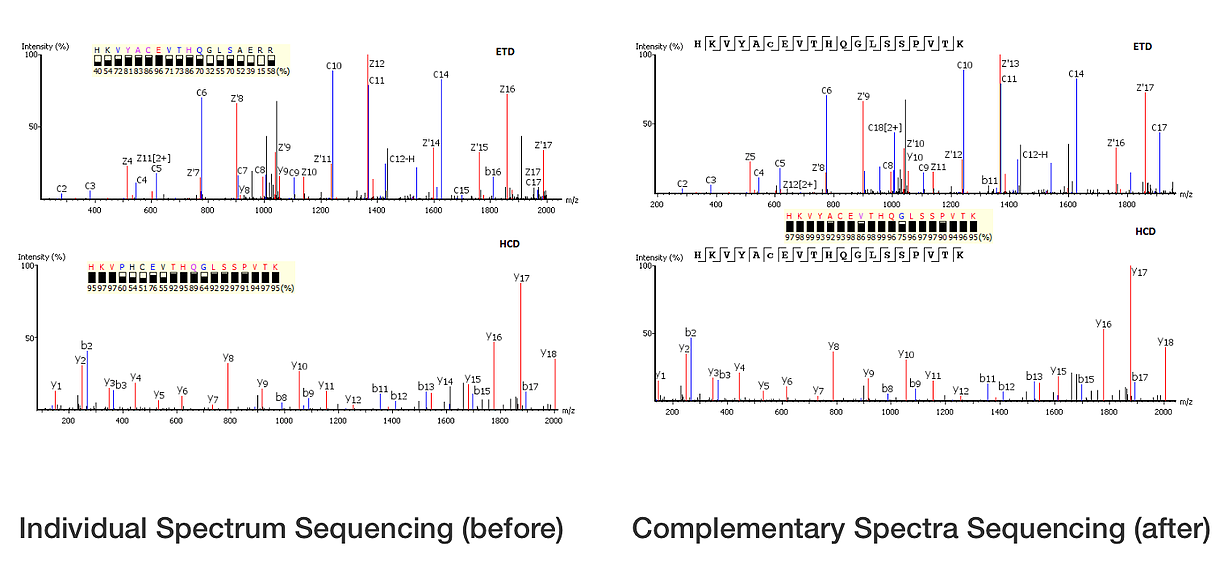
Although various machine learning methods have been developed to address this de novo sequencing problem, challenges that arise when modeling tandem mass spectra have led to complex models that combine multiple neural networks and post-processing steps. A key outstanding challenge in this field involves identifying the sequence of amino acids-the peptide-responsible for generating each observed spectrum, without making use of prior knowledge in the form of a peptide sequence database. Tandem mass spectrometry is the only high-throughput method for analyzing the protein content of complex biological samples and is thus the primary technology driving the growth of the field of proteomics.
